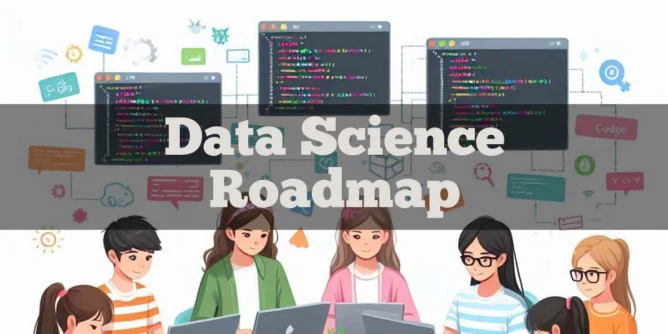
A Data Science Roadmap outlines the necessary skills, tools, and technologies to become a proficient data scientist. Here’s a structured roadmap that will guide you through the essential stages of learning and mastering data science:
1. Foundation: Basic Skills
a. Mathematics & Statistics
Linear Algebra: Vectors, matrices, eigenvalues, and eigenvectors. Fundamental for understanding machine learning algorithms.
Probability & Statistics:
Probability theory, distributions (normal, binomial, etc.), and Bayes’ theorem.
Statistical tests, hypothesis testing, p-values, confidence intervals.
Regression analysis, correlation, and data distribution understanding.
b. Programming Languages
Python: The most widely-used language for data science due to its rich libraries and versatility.
Key Libraries:
NumPy
,Pandas
(data manipulation),Matplotlib
,Seaborn
(data visualization),SciPy
(scientific computing),Scikit-learn
(machine learning).
R: Another popular language for statistical analysis, especially in academia and research.
c. Data Manipulation & Analysis
Data Cleaning: Handling missing data, outliers, duplicates.
Data Wrangling: Merging, reshaping, and transforming datasets.
Exploratory Data Analysis (EDA): Understand your data using descriptive statistics and visualizations.
2. Intermediate Skills: Core Data Science Tools
a. SQL (Structured Query Language)
Data Querying: Learn how to retrieve and manipulate data from relational databases.
Key Concepts:
SELECT
,JOIN
,WHERE
,GROUP BY
,ORDER BY
, subqueries, and window functions.
b. Data Visualization Tools
Tableau or Power BI: For creating advanced, interactive dashboards and data visualizations.
Matplotlib, Seaborn (Python) or ggplot2 (R) for more customizable and static visualizations.
c. Machine Learning Fundamentals
Supervised Learning: Understand classification and regression models (e.g., linear regression, decision trees, support vector machines).
Unsupervised Learning: Learn clustering techniques (e.g., K-means, hierarchical clustering).
Model Evaluation: Learn key evaluation metrics such as accuracy, precision, recall, F1 score, ROC curve, and AUC.
d. Big Data Technologies
Hadoop: Learn the basics of Hadoop for distributed storage and processing.
Spark: For big data analysis and machine learning on large datasets.
3. Advanced Skills: In-Depth Knowledge and Specialization
a. Advanced Machine Learning
Ensemble Methods: Random Forest, Gradient Boosting, and XGBoost.
Deep Learning: Neural networks, backpropagation, CNNs (Convolutional Neural Networks), and RNNs (Recurrent Neural Networks) using frameworks like TensorFlow or PyTorch.
Natural Language Processing (NLP): Text analysis, sentiment analysis, and working with text data using libraries like NLTK and spaCy.
Reinforcement Learning: Learn how agents make decisions in dynamic environments.
b. Data Engineering Skills (Optional but Helpful)
ETL (Extract, Transform, Load): Learn how to build and manage data pipelines.
Cloud Platforms: Familiarize yourself with cloud services such as AWS, Google Cloud, or Azure for managing large-scale data infrastructure.
c. Advanced Statistics & Modeling
Bayesian Inference: Learn how to incorporate prior knowledge into your models.
Time Series Analysis: Work with sequential data and forecast future values.
Optimization Algorithms: Learn about gradient descent and other optimization techniques used in machine learning.
4. Specialized Skills: Mastery in Data Science
a. Deep Learning & AI
Neural Networks: Learn architectures like Multi-Layer Perceptrons (MLPs), Convolutional Neural Networks (CNNs), and Recurrent Neural Networks (RNNs).
AI & Automation: Automate decision-making using AI models, deep reinforcement learning, and advanced optimization.
b. Business & Communication Skills
Business Acumen: Learn to identify business problems that can be solved with data science and analytics.
Communication: Ability to translate complex technical findings into actionable insights for non-technical stakeholders.
Reporting: Create clear and effective reports and presentations using data visualizations and storytelling techniques.
5. Professional Development
a. Build a Portfolio
Personal Projects: Work on real-world data problems, Kaggle competitions, or open-source contributions to showcase your skills.
GitHub: Maintain a public repository of your projects, algorithms, and notebooks.
b. Certifications
Google Data Analytics Professional Certificate
IBM Data Science Professional Certificate
Microsoft Certified: Azure Data Scientist
Deep Learning Specialization by Andrew Ng (Coursera)
c. Networking
Attend data science conferences like Strata Data, PyData, or local meetups.
Engage with the data science community on platforms like Kaggle, Stack Overflow, and LinkedIn.
6. Job Market & Career Growth
a. Entry-Level Data Scientist Role
Skills: Strong Python skills, statistical knowledge, basic machine learning, and SQL.
Responsibilities: Data cleaning, EDA, simple model building, data visualization.
b. Mid-Level Data Scientist
Skills: Expertise in machine learning algorithms, proficiency in tools like TensorFlow/PyTorch, and hands-on experience with big data tools like Spark.
Responsibilities: Building and deploying machine learning models, creating advanced visualizations, interpreting model results.
c. Senior Data Scientist / AI Specialist
Skills: Deep knowledge of advanced machine learning, AI, and data engineering.
Responsibilities: Leading data science teams, designing complex machine learning systems, integrating data science into business strategy.
7. Stay Updated
Data science evolves rapidly. Keep learning:
Read industry blogs, follow researchers and practitioners on social media, and subscribe to journals.
Participate in data science competitions on Kaggle and engage with the community.
This roadmap provides a clear, progressive path from mastering foundational skills to advanced specializations in data science. By following it, you can build a strong career in the data science field, adapting to both the growing demand for technical expertise and business-oriented insights.